Innovating through Murray–Darling Basin challenges
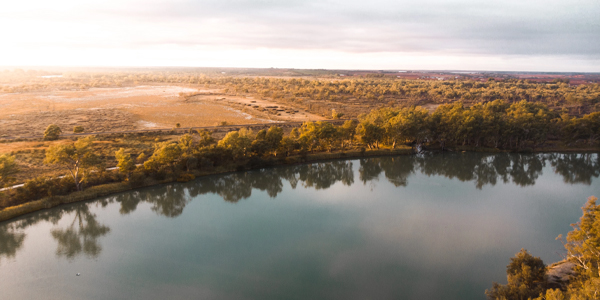
With climate change impacting uncertainties in water availability, the Murray–Darling Basin faces mounting pressure to adapt. Researchers working with the One Basin CRC are addressing these challenges by pioneering innovative methods, transforming how we plan for climate resilience despite the unpredictable nature of future climate impacts.
In a recent webinar on One Basin CRC's project on climate risk management in the Murray–Darling Basin, University of Melbourne’s Professor Avril Horne and Dr Andrew John presented on new methods for stress testing climate impacts on water resources.
Hosting the online event, ANU Institute for Water Futures Research Fellow and One Basin CRC’s Foresight and Decisions program lead, Dr Joseph Guillaume said the project is one of the One Basin CRC’s earliest contributions demonstrating how the CRC can help transform how we look at climate risk.
“We have an opportunity here to support planning that is not just optimised for a likely future, but resilient to a wide range of futures,” he said.
"This project is part of Australia's contribution to a global transformation, which is also about better understanding how our highly modified landscape works under different conditions, rather than aiming to stay in control at all times."
The One Basin CRC is an industry and research collaboration aiming to solve significant and complex water-related challenges in the Murray–Darling Basin, bringing together 85 partner organisations from around Australia.
"[The project] will help government and industry and communities prepare for a different future by building our understanding of what the future could look like, providing the information, tools, and resources required to manage climate and water-related risk,” Guillaume said.
How does stress testing work in water management?
When it comes to managing water challenges in the future, Associate Professor Avril Horne said stress testing for climate resilience is an approach that’s significantly different from traditional ways of assessing risk.
"We know from research that faced with this level of uncertainty, many people struggle to make decisions and instead want to wait for more information or to see how things unfold,” she said.
"This project is about stress testing, asking: can our system cope with the full range of plausible futures and are there management interventions that increase the robustness of our system to that range?
"Stress testing flips on its head the way that we’ve traditionally thought about doing climate impact assessments. Traditional methods are top-down, starting with global climate models and working towards outcomes.
“Stress testing instead begins with the objectives we care about and tests how far we can stress the system before those objectives fail."
For the project, researchers generated stochastic climate-impacted flow across the entire Murray–Darling Basin, exploring rapid modeling methods to balance precision, speed and flexibility to incorporate uncertainty into system analysis.
The researchers first stress-tested water allocations across the southern Basin: "The allocation modeling process revealed the sensitivity differences between river systems and how allocation policies distribute climate impacts differently,” Horne said.
“For instance, New South Wales allocates water as it becomes available, whereas Victoria has a process that socialises some of that risk.”
These differences in the allocation policies mean that water in Victoria and NSW have different levels of resilience. NSW allocations respond gradually as the climate changes, whereas Victoria can rapidly degrade beyond a certain degree of climate change.
"Using stress testing, we can create a vulnerability surface, a tool that explains how systems respond to incremental climate changes. It also helps identify thresholds, points where performance drops rapidly, allowing us to understand where management interventions are needed,” Horne said.
"We’ve shown it’s possible to rapidly generate monthly stochastic data for stress testing across a large river catchment. This process, now available and transferable to other systems, has been pivotal in understanding the robustness of our models."
Adopting this process is about improving our understanding of the system and helping inform scenario selection, Horne said.
"Ultimately, these methods improve knowledge of vulnerability and resilience, offering robust adaptation options that function despite future uncertainties,” she said.
Machine learning for complex water systems
A major challenge in addressing climate change uncertainty in the Basin lies in the significant time and cost required to operate current complex river models.
Outlining how machine learning simplifies water system modeling by replacing parts of regulated systems, Dr Andrew John said that by training emulator models on outputs of complex resource models, efficient, accurate insights into system behavior under diverse climate scenarios can be achieved.
John said these emulator approaches can complement existing complex models by allowing assessment of robustness and resilience.
"When we trained emulator models on long sequences of existing water resource model outcomes with various climate scenarios, they mimicked the complex models quite well,” he said.
"We found that machine learning models, when trained with additional climate scenarios for variability, performed remarkably well at projecting flows into different situations they hadn’t encountered before.
"We found the northern Basin valleys tended to be about twice as sensitive to changes in precipitation and increases in temperature compared to the southern basin."
Using stress testing, researchers plotted thresholds for various hydrologic metrics, showing where reductions in flow components occur.
John said low flow indicators, which are more actively controlled by existing regulation infrastructure, often showed different thresholds for change compared to high flows.
“This suggests that adaptation is easier for low flows but far more challenging for high flows,” he said.
"The Murray barrage indicators showed interesting behavior, tracking well until about a 10% reduction in precipitation, after which performance dropped rapidly.”
"These approaches are useful for learning about system behavior and finding concerning thresholds where outcomes start to degrade rapidly under specific conditions. This knowledge helps pinpoint potential system failures before they occur,” John said.
"We can simulate adaptation responses or management changes quickly by retraining the machine learning models for new scenarios. This flexibility is key to exploring how the system might respond to different interventions.
"Ultimately, this work supports developing high-impact planning scenarios that test whether we can pull the system away from failure under a range of future conditions, helping inform resilient and adaptive management strategies."
Interested in learning more about One Basin CRC research projects? Take a look here.