How machine learning could improve response to infrastructure needs
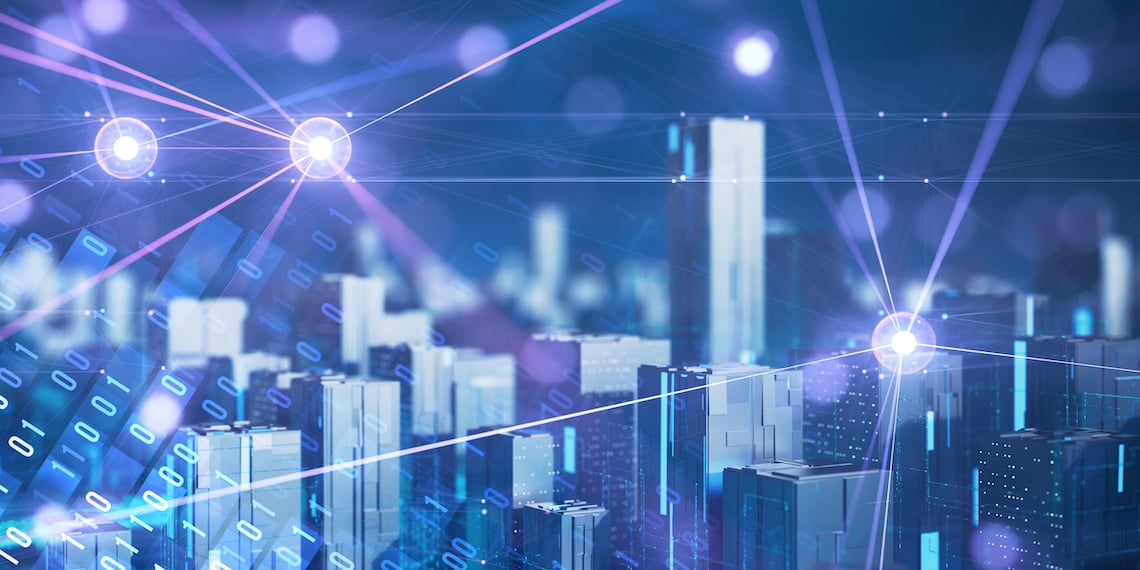
Researchers in South East Queensland hope to make land use and water infrastructure smarter and more effective with the help of machine learning algorithms.
Griffith University PhD student and Senior Urban Analytics Engineer at UACS Consulting, Abhijeet Dashwant, who is set to present on the research at Ozwater’23 in May, said that while many utilities use machine learning for activities such as leak detection, the technology has more to offer.
Dashwant’s research project will seek to bring together land use and infrastructure planning using a more dynamic, integrated approach.
“During the planning process, not all decisions are made efficiently, because there are no supporting tools that give evidence-based results to inform policy makers or politicians so they can make decisions,” Dashwant said.
“It's not that current approaches aren’t efficient; it's just that there is a lot of complexity involved.”
While Dashwant cautions that his research is in its early stages and is yet to go through peer review, he hopes his algorithms will one day be able to help cut through that complexity, modelling potential changes so that planners can better understand the implications.
“The model developed through my PhD project seeks to use machine-learning algorithms to remove the complexity involved in the process, and potentially assist planners and modellers to better understand the implications of the choices they apply to solve urban development challenges,” Dashwant said.
“Current approaches use a manual trial-and-error approach that takes longer model runtimes, which affects the decision process. With the current approach, it can get more resource intensive to make the process faster.”
The project work is a collaborative effort with the Queensland Government and local governments in South East Queensland.
The model Dashwant is developing will seek to inform governments’ decision-making processes, helping to better plan for growth and make their land use and infrastructure development more time and resource efficient.
Model planning
Dashwant’s work so far has consisted of a preliminary analysis that uses a benchmark model named “D-Town,” which was originally developed for an Adelaide water distribution system analysis in 2012, and can be used to understand and solve traditional water network problems.
“It's a benchmark model that water researchers use to see whether our concept will work,” Dashwant explained.
“Dummy growth scenarios were used to test the project concept to see what the model would predict, and it showed positive results. It was predicting upgrades which were required based on the growth and capital-cost calculations.”
The model takes into account both water and sewer networks in its calculations.
“By using both water and sewer data, and then using algorithmic techniques, you can apply the optimisation part,” Dashwant said.
“Optimisation algorithms require: decision variables, including pipes, pumps or valves; constraints, including hydraulic and system; and objectives – economic, community and environmental – to be defined beforehand to run algorithms.
“Genetic algorithms are the most popular algorithms used for this purpose. Recent trends in machine learning are showing confidence in achieving better and faster solutions.”
Using machine learning in this way also means complex and larger network problems have the potential to be solved.
“Machine learning requires a large dataset for model training and makes better predictions,” Dashwant said.
Advancing technology
Dashwant expects to have results from the research next year and is considering what other uses a machine-learning approach might have for councils.
“An integrated planning approach is usually in the strategy goals of a water utility,” he said.
“This project intends to demonstrate an integrated planning approach for water and sewerage, but other infrastructure types will need to be considered, such as transport and stormwater.”
Dashwant said it helps that machine-learning technology is constantly improving.
“The technology is advancing quickly and utilities want to know how they can apply this rapidly changing technology to benefit the business,” he said.
“Hopefully, there will be more projects coming to make systems work more efficiently for decision makers.
“I'm getting more and more positive feedback about this and they're so excited about the progress of this project. There's definitely a strong interest in this work.”
Interested in hearing more about what Dashwant’s work with machine learning can do to improve water infrastructure? Register for Ozwater’23 here.